ValueError: Cannot set tensor: Dimension mismatch. Got 3 but expected 4 for input 0(ValueError:无法设置张量:维度不匹配。已获取%3,但输入%0应为%4)
本文介绍了ValueError:无法设置张量:维度不匹配。已获取%3,但输入%0应为%4的处理方法,对大家解决问题具有一定的参考价值,需要的朋友们下面随着小编来一起学习吧!
问题描述
我是特遣部队和凯拉斯的新手。我已经使用以下代码对模型进行了培训和保存
from tensorflow.keras.preprocessing.image import ImageDataGenerator
import tensorflow as tf
from tensorflow.python.keras.optimizer_v2.rmsprop import RMSprop
train_data_gen = ImageDataGenerator(rescale=1 / 255)
validation_data_gen = ImageDataGenerator(rescale=1 / 255)
# Flow training images in batches of 120 using train_data_gen generator
train_generator = train_data_gen.flow_from_directory(
'datasets/train/',
classes=['bad', 'good'],
target_size=(200, 200),
batch_size=120,
class_mode='binary')
validation_generator = validation_data_gen.flow_from_directory(
'datasets/valid/',
classes=['bad', 'good'],
target_size=(200, 200),
batch_size=19,
class_mode='binary',
shuffle=False)
model = tf.keras.models.Sequential([
tf.keras.layers.Conv2D(16, (3, 3), activation='relu', input_shape=(200, 200, 3)),
tf.keras.layers.MaxPooling2D(2, 2),
tf.keras.layers.Conv2D(32, (3, 3), activation='relu'),
tf.keras.layers.MaxPooling2D(2, 2),
tf.keras.layers.Conv2D(64, (3, 3), activation='relu'),
tf.keras.layers.MaxPooling2D(2, 2),
tf.keras.layers.Conv2D(64, (3, 3), activation='relu'),
tf.keras.layers.MaxPooling2D(2, 2),
tf.keras.layers.Conv2D(64, (3, 3), activation='relu'),
tf.keras.layers.MaxPooling2D(2, 2),
# Flatten the results to feed into a DNN
tf.keras.layers.Flatten(),
# 512 neuron hidden layer
tf.keras.layers.Dense(512, activation='relu'),
# Only 1 output neuron. It will contain a value from 0-1
# where 0 for 1 class ('bad') and 1 for the other ('good')
tf.keras.layers.Dense(1, activation='sigmoid')])
model.compile(loss='binary_crossentropy',
optimizer=RMSprop(lr=0.001),
metrics='accuracy')
model.fit(train_generator,
steps_per_epoch=10,
epochs=25,
verbose=1,
validation_data=validation_generator,
validation_steps=8)
print("Evaluating the model :")
model.evaluate(validation_generator)
print("Predicting :")
validation_generator.reset()
predictions = model.predict(validation_generator, verbose=1)
print(predictions)
model.save("models/saved")
然后使用
将此模型转换为tfliteimport tensorflow as tf
def saved_model_to_tflite(model_path, quantize):
converter = tf.lite.TFLiteConverter.from_saved_model(model_path)
model_saving_path = "models/converted/model.tflite"
if quantize:
converter.optimizations = [tf.lite.Optimize.DEFAULT]
model_saving_path = "models/converted/model-quantized.tflite"
tflite_model = converter.convert()
with open(model_saving_path, 'wb') as f:
f.write(tflite_model)
然后使用
针对单个图像测试模型import tensorflow as tf
def run_tflite_model(tflite_file, test_image):
interpreter = tf.lite.Interpreter(model_path=str(tflite_file))
interpreter.allocate_tensors()
print(interpreter.get_input_details())
input_details = interpreter.get_input_details()[0]
output_details = interpreter.get_output_details()[0]
interpreter.set_tensor(input_details["index"], test_image)
interpreter.invoke()
output = interpreter.get_tensor(output_details["index"])[0]
prediction = output.argmax()
return prediction
main.py
if __name__ == '__main__':
converted_model = "models/converted/model.tflite"
bad_image_path = "datasets/experiment/bad/b.png"
good_image_path = "datasets/experiment/good/g.png"
img = io.imread(bad_image_path)
resized = resize(img, (200, 200)).astype('float32')
prediction = run_tflite_model(converted_model, resized)
print(prediction)
但即使我将图像大小调整为200 x 200
ValueError: Cannot set tensor: Dimension mismatch. Got 3 but expected 4 for input 0.
如果我这样做print(interpreter.get_input_details())
[{'name': 'serving_default_conv2d_input:0', 'index': 0, 'shape': array([ 1, 200, 200, 3], dtype=int32), 'shape_signature': array([ -1, 200, 200, 3], dtype=int32), 'dtype': <class 'numpy.float32'>, 'quantization': (0.0, 0), 'quantization_parameters': {'scales': array([], dtype=float32), 'zero_points': array([], dtype=int32), 'quantized_dimension': 0}, 'sparsity_parameters': {}}]
所以输入的形状似乎是'shape': array([ 1, 200, 200, 3]
我确实得到了部件200, 200, 3
似乎1
批次大小是根据docs?
如何从输入形状中删除批处理大小?
推荐答案
您可以使用EXPAND_DIMS:
来展开维,而不是删除图形中的批处理大小test_image = np.expand_dims(test_image, axis=0)
对于Android,您可以通过循环复制值,轻松地从Float[32][32][3]输入数组准备Float[1][32][32][3]输入数组。
这篇关于ValueError:无法设置张量:维度不匹配。已获取%3,但输入%0应为%4的文章就介绍到这了,希望我们推荐的答案对大家有所帮助,也希望大家多多支持编程学习网!
沃梦达教程
本文标题为:ValueError:无法设置张量:维度不匹配。已获取%3,但输入%0应为%4
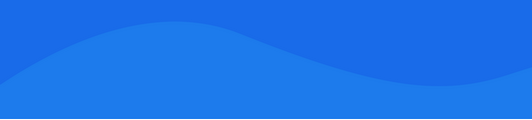

基础教程推荐
猜你喜欢
- Python 的 List 是如何实现的? 2022-01-01
- 合并具有多索引的两个数据帧 2022-01-01
- 使用 Google App Engine (Python) 将文件上传到 Google Cloud Storage 2022-01-01
- 哪些 Python 包提供独立的事件系统? 2022-01-01
- 症状类型错误:无法确定关系的真值 2022-01-01
- 使用Python匹配Stata加权xtil命令的确定方法? 2022-01-01
- 如何在 Python 中检测文件是否为二进制(非文本)文 2022-01-01
- 如何在Python中绘制多元函数? 2022-01-01
- 使 Python 脚本在 Windows 上运行而不指定“.py";延期 2022-01-01
- 将 YAML 文件转换为 python dict 2022-01-01