Definitive way to match Stata weighted xtile command using Python?(使用Python匹配Stata加权xtil命令的确定方法?)
问题描述
对于一个项目,我需要复制一些结果,这些结果当前存在于Stata输出文件(.dta)中,并且是从较旧的Stata脚本计算得出的。项目的新版本需要用Python语言编写。
我遇到困难的具体部分是匹配基于Stataxtile
command加权版本的分位数断点计算。请注意,数据点之间的关联与权重无关,而我使用的权重来自连续的数量,因此关联是极不可能的(并且我的测试数据集中没有关联)。因此,由于联系而导致的分类错误并不是问题所在。
我已阅读Wikipedia article on weighted percentiles和this Cross-Validated post,其中介绍了应复制R的第7类分位数的替代算法。
我已经实现了这两个加权算法(底部的代码),但我仍然不能很好地匹配Stata输出中计算的分位数。
有人知道Stata例程使用的特定算法吗?文件没有明确描述这一点。它说的是在CDF的平坦部分取平均值来倒置它,但这几乎不能描述实际的算法,并且不清楚它是否正在进行任何其他内插。
请注意,numpy.percentile
和scipy.stats.mstats.mquantiles
不接受权重,也不能执行加权分位数,只能执行常规的等权分位数。我的问题的症结在于需要使用权重。
注意:我已经对下面的两种方法进行了相当多的调试,但如果您看到一个错误,请在评论中提出错误建议。我已经在较小的数据集上测试了这两种方法,结果很好,而且在我可以保证R使用的是什么方法的情况下,也符合R的输出。代码还不是很优雅,在两个类型之间复制了太多内容,但当我相信输出是我所需要的时,所有这些都会在稍后得到修复。
问题是我不知道Stataxtile
使用的方法,我希望减少下面的代码与在相同数据集上运行的Stataxtile
之间的不匹配。
我尝试过的算法:
import numpy as np
def mark_weighted_percentiles(a, labels, weights, type):
# a is an input array of values.
# weights is an input array of weights, so weights[i] goes with a[i]
# labels are the names you want to give to the xtiles
# type refers to which weighted algorithm.
# 1 for wikipedia, 2 for the stackexchange post.
# The code outputs an array the same shape as 'a', but with
# labels[i] inserted into spot j if a[j] falls in x-tile i.
# The number of xtiles requested is inferred from the length of 'labels'.
# First type, "vanilla" weights from Wikipedia article.
if type == 1:
# Sort the values and apply the same sort to the weights.
N = len(a)
sort_indx = np.argsort(a)
tmp_a = a[sort_indx].copy()
tmp_weights = weights[sort_indx].copy()
# 'labels' stores the name of the x-tiles the user wants,
# and it is assumed to be linearly spaced between 0 and 1
# so 5 labels implies quintiles, for example.
num_categories = len(labels)
breaks = np.linspace(0, 1, num_categories+1)
# Compute the percentile values at each explicit data point in a.
cu_weights = np.cumsum(tmp_weights)
p_vals = (1.0/cu_weights[-1])*(cu_weights - 0.5*tmp_weights)
# Set up the output array.
ret = np.repeat(0, len(a))
if(len(a)<num_categories):
return ret
# Set up the array for the values at the breakpoints.
quantiles = []
# Find the two indices that bracket the breakpoint percentiles.
# then do interpolation on the two a_vals for those indices, using
# interp-weights that involve the cumulative sum of weights.
for brk in breaks:
if brk <= p_vals[0]:
i_low = 0; i_high = 0;
elif brk >= p_vals[-1]:
i_low = N-1; i_high = N-1;
else:
for ii in range(N-1):
if (p_vals[ii] <= brk) and (brk < p_vals[ii+1]):
i_low = ii
i_high = ii + 1
if i_low == i_high:
v = tmp_a[i_low]
else:
# If there are two brackets, then apply the formula as per Wikipedia.
v = tmp_a[i_low] + ((brk-p_vals[i_low])/(p_vals[i_high]-p_vals[i_low]))*(tmp_a[i_high]-tmp_a[i_low])
# Append the result.
quantiles.append(v)
# Now that the weighted breakpoints are set, just categorize
# the elements of a with logical indexing.
for i in range(0, len(quantiles)-1):
lower = quantiles[i]
upper = quantiles[i+1]
ret[ np.logical_and(a>=lower, a<upper) ] = labels[i]
#make sure upper and lower indices are marked
ret[a<=quantiles[0]] = labels[0]
ret[a>=quantiles[-1]] = labels[-1]
return ret
# The stats.stackexchange suggestion.
elif type == 2:
N = len(a)
sort_indx = np.argsort(a)
tmp_a = a[sort_indx].copy()
tmp_weights = weights[sort_indx].copy()
num_categories = len(labels)
breaks = np.linspace(0, 1, num_categories+1)
cu_weights = np.cumsum(tmp_weights)
# Formula from stats.stackexchange.com post.
s_vals = [0.0];
for ii in range(1,N):
s_vals.append( ii*tmp_weights[ii] + (N-1)*cu_weights[ii-1])
s_vals = np.asarray(s_vals)
# Normalized s_vals for comapring with the breakpoint.
norm_s_vals = (1.0/s_vals[-1])*s_vals
# Set up the output variable.
ret = np.repeat(0, N)
if(N < num_categories):
return ret
# Set up space for the values at the breakpoints.
quantiles = []
# Find the two indices that bracket the breakpoint percentiles.
# then do interpolation on the two a_vals for those indices, using
# interp-weights that involve the cumulative sum of weights.
for brk in breaks:
if brk <= norm_s_vals[0]:
i_low = 0; i_high = 0;
elif brk >= norm_s_vals[-1]:
i_low = N-1; i_high = N-1;
else:
for ii in range(N-1):
if (norm_s_vals[ii] <= brk) and (brk < norm_s_vals[ii+1]):
i_low = ii
i_high = ii + 1
if i_low == i_high:
v = tmp_a[i_low]
else:
# Interpolate as in the type 1 method, but using the s_vals instead.
v = tmp_a[i_low] + (( (brk*s_vals[-1])-s_vals[i_low])/(s_vals[i_high]-s_vals[i_low]))*(tmp_a[i_high]-tmp_a[i_low])
quantiles.append(v)
# Now that the weighted breakpoints are set, just categorize
# the elements of a as usual.
for i in range(0, len(quantiles)-1):
lower = quantiles[i]
upper = quantiles[i+1]
ret[ np.logical_and( a >= lower, a < upper ) ] = labels[i]
#make sure upper and lower indices are marked
ret[a<=quantiles[0]] = labels[0]
ret[a>=quantiles[-1]] = labels[-1]
return ret
推荐答案
以下是STATA 12手册(STATA Corp.2011年。STATA统计软件:Release 12.College Station,TX:StataCorp LP,p.501-502)。如果这不起作用,您可以在Statist上问这个问题,或者直接联系Philip Ryan(原始代码的作者)。
这篇关于使用Python匹配Stata加权xtil命令的确定方法?的文章就介绍到这了,希望我们推荐的答案对大家有所帮助,也希望大家多多支持编程学习网!
本文标题为:使用Python匹配Stata加权xtil命令的确定方法?
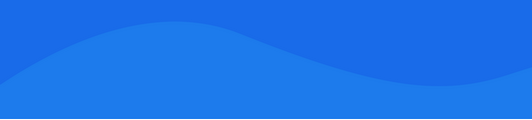

基础教程推荐
- 将 YAML 文件转换为 python dict 2022-01-01
- 使用Python匹配Stata加权xtil命令的确定方法? 2022-01-01
- 如何在Python中绘制多元函数? 2022-01-01
- 如何在 Python 中检测文件是否为二进制(非文本)文 2022-01-01
- 哪些 Python 包提供独立的事件系统? 2022-01-01
- Python 的 List 是如何实现的? 2022-01-01
- 使用 Google App Engine (Python) 将文件上传到 Google Cloud Storage 2022-01-01
- 使 Python 脚本在 Windows 上运行而不指定“.py";延期 2022-01-01
- 合并具有多索引的两个数据帧 2022-01-01
- 症状类型错误:无法确定关系的真值 2022-01-01