power spectrum by numpy.fft.fft(基于numpy.fft.fft的功率谱)
本文介绍了基于numpy.fft.fft的功率谱的处理方法,对大家解决问题具有一定的参考价值,需要的朋友们下面随着小编来一起学习吧!
问题描述
我通过下面的代码绘制的数字只是零附近的峰值,无论我如何更改数据。我的数据只是一列,它记录了某种信号的每个定时点。time_step
是我应该根据数据中两个邻接点的间隔定义的值吗?
data=np.loadtxt("timesequence",delimiter=",",usecols=(0,),unpack=True)
ps = np.abs(np.fft.fft(data))**2
time_step = 1
freqs = np.fft.fftfreq(data.size, time_step)
idx = np.argsort(freqs)
pl.plot(freqs[idx], ps[idx])
pl.show()
推荐答案
正如其他人所暗示的,您的信号必须具有较大的非零分量。0(DC)处的峰值表示信号的平均值。这是从傅里叶变换本身导出的。该余弦函数cos(0)*ps(0)表示信号平均值的度量。其他傅立叶变换分量是振幅可变的余弦波,它们在这些值上显示频率内容。
请注意,平稳信号将不具有大的DC分量,因为它们已经是零均值信号。如果你不想要很大的直流分量,那么你应该计算信号的平均值并从中减去数值。无论您的数据是0,.,999还是1,.,1000,甚至1000,.,2000,您都会在0 HZ处达到峰值。唯一的区别将是峰值的大小,因为它测量的是平均值。data1 = arange(1000)
data2 = arange(1000)+1000
dataTransformed3 = data - mean(data)
data4 = numpy.zeros(1000)
data4[::10] = 1 #simulate a photon counter where a 1 indicates a photon came in at time indexed by array.
# we could assume that the sample rate was 10 Hz for example
ps1 = np.abs(np.fft.fft(data))**2
ps2 = np.abs(np.fft.fft(data))**2
ps3 = np.abs(np.fft.fft(dataTransformed))**2
figure()
plot(ps1) #shows the peak at 0 Hz
figure()
plot(ps2) #shows the peak at 0 Hz
figure()
plot(ps3) #shows the peak at 1 Hz this is because we removed the mean value but since
#the function is a step function the next largest component is the 1 Hz cosine wave.
#notice the order of magnitude difference in the two plots.
这篇关于基于numpy.fft.fft的功率谱的文章就介绍到这了,希望我们推荐的答案对大家有所帮助,也希望大家多多支持编程学习网!
沃梦达教程
本文标题为:基于numpy.fft.fft的功率谱
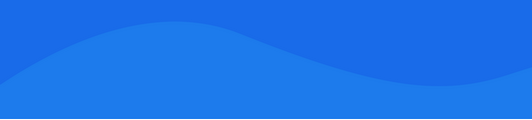

基础教程推荐
猜你喜欢
- Python 的 List 是如何实现的? 2022-01-01
- 使用 Google App Engine (Python) 将文件上传到 Google Cloud Storage 2022-01-01
- 如何在 Python 中检测文件是否为二进制(非文本)文 2022-01-01
- 使 Python 脚本在 Windows 上运行而不指定“.py";延期 2022-01-01
- 哪些 Python 包提供独立的事件系统? 2022-01-01
- 将 YAML 文件转换为 python dict 2022-01-01
- 症状类型错误:无法确定关系的真值 2022-01-01
- 如何在Python中绘制多元函数? 2022-01-01
- 合并具有多索引的两个数据帧 2022-01-01
- 使用Python匹配Stata加权xtil命令的确定方法? 2022-01-01