Sklearn and StatsModels give very different logistic regression answers(SkLearning和StatsModels给出了截然不同的Logistic回归答案)
本文介绍了SkLearning和StatsModels给出了截然不同的Logistic回归答案的处理方法,对大家解决问题具有一定的参考价值,需要的朋友们下面随着小编来一起学习吧!
问题描述
我正在对布尔0/1数据集进行Logistic回归(预测某个年龄超过某个金额的工资的概率),并且我使用sklearn和StatsModels得到了非常不同的结果,而skLearning是非常错误的。
为了使该函数更类似于StatsModels,我已将skLearning惩罚设置为None,并将Intercept Term设置为False,但我看不到如何让skLearning给出合理的答案。
灰色线条是位于0或1处的原始数据点,我刚刚将绘图上的1缩小到0.1才可见。
变量:
# X and Y
X = df.age.values.reshape(-1,1)
X_poly = PolynomialFeatures(degree=4).fit_transform(X)
y_bool = np.array(df.wage.values > 250, dtype = "int")
# Generate a sequence of ages
age_grid = np.arange(X.min(), X.max()).reshape(-1,1)
age_grid_poly = PolynomialFeatures(degree=4).fit_transform(age_grid)
代码如下:
# sklearn Model
clf = LogisticRegression(penalty = None, fit_intercept = False,max_iter = 300).fit(X=X_poly, y=y_bool)
preds = clf.predict_proba(age_grid_poly)
# Plot
fig, ax = plt.subplots(figsize=(8,6))
ax.scatter(X ,y_bool/10, s=30, c='grey', marker='|', alpha=0.7)
plt.plot(age_grid, preds[:,1], color = 'r', alpha = 1)
plt.xlabel('Age')
plt.ylabel('Wage')
plt.show()
sklearn result
# StatsModels
log_reg = sm.Logit(y_bool, X_poly).fit()
preds = log_reg.predict(age_grid_poly)
# Plot
fig, ax = plt.subplots(figsize=(8,6))
ax.scatter(X ,y_bool/10, s=30, c='grey', marker='|', alpha=0.7)
plt.plot(age_grid, preds, color = 'r', alpha = 1)
plt.xlabel('Age')
plt.ylabel('Wage')
plt.show()
StatsModels result
推荐答案
这似乎是因为SkLearning的实现非常依赖于规模(而且多项式项非常大)。通过首先对数据进行缩放,我得到的结果在质量上是相同的。
# sklearn Model
from sklearn.preprocessing import StandardScaler
from sklearn.pipeline import Pipeline
clf = Pipeline([
('scale', StandardScaler()),
('lr', LogisticRegression(penalty='none', fit_intercept=True, max_iter=1000)),
]).fit(X=X_poly, y=y_bool)
preds = clf.predict_proba(age_grid_poly)
# Plot
fig, ax = plt.subplots(figsize=(8,6))
ax.scatter(X ,y_bool/10, s=30, c='grey', marker='|', alpha=0.7)
plt.plot(age_grid, preds[:,1], color = 'r', alpha = 1)
plt.xlabel('Age')
plt.ylabel('Wage')
plt.show()
请注意,在本例中我们需要设置fit_intercept=True
,因为StandardScaler
会删除来自PolynomialFeatures
的常量列(使其全为零)。
这篇关于SkLearning和StatsModels给出了截然不同的Logistic回归答案的文章就介绍到这了,希望我们推荐的答案对大家有所帮助,也希望大家多多支持编程学习网!
沃梦达教程
本文标题为:SkLearning和StatsModels给出了截然不同的Logistic回归答案
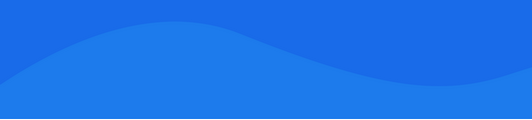

基础教程推荐
猜你喜欢
- 使 Python 脚本在 Windows 上运行而不指定“.py";延期 2022-01-01
- 将 YAML 文件转换为 python dict 2022-01-01
- 症状类型错误:无法确定关系的真值 2022-01-01
- Python 的 List 是如何实现的? 2022-01-01
- 如何在 Python 中检测文件是否为二进制(非文本)文 2022-01-01
- 合并具有多索引的两个数据帧 2022-01-01
- 使用Python匹配Stata加权xtil命令的确定方法? 2022-01-01
- 哪些 Python 包提供独立的事件系统? 2022-01-01
- 如何在Python中绘制多元函数? 2022-01-01
- 使用 Google App Engine (Python) 将文件上传到 Google Cloud Storage 2022-01-01