Computing Jaccard similarity on multiple dictionaries in Python?(在Python中计算多个词典上的Jaccard相似度?)
本文介绍了在Python中计算多个词典上的Jaccard相似度?的处理方法,对大家解决问题具有一定的参考价值,需要的朋友们下面随着小编来一起学习吧!
问题描述
我有一本词典是这样的:
my_dict = {'Community A': ['User 1', 'User 2', 'User 3'],
'Community B': ['User 1', 'User 2'],
'Community C': ['User 3', 'User 4', 'User 5'],
'Community D': ['User 1', 'User 3', 'User 4', 'User 5']}
我的目标是对不同社区及其独特用户集之间的网络关系进行建模,以查看哪些社区最相似。目前,我正在探索使用Jaccard相似性。
我遇到过执行类似操作的答案,但只在两本词典上;在我的情况下,我有几本词典,需要计算每组词典之间的相似度。
另外,有些列表的长度不同:在其他答案中,我将0
subin视为该情况下的缺失值,我认为这在我的情况下是可行的。
推荐答案
您需要的是Jaccard相似性矩阵。如果您希望通过元组(GroupA、GroupB)进行索引,则可以将它们存储为词典。
下面是一个简单的实现
def jaccard(first, second):
return len(set(first).intersection(second)) / len(set(first).union(second))
keys = list(my_dict.keys())
result_dict = {}
for k in keys:
for l in keys:
result_dict[(k,l)] = result_dict.get((l,k), jaccard(my_dict[k], my_dict[l]))
则结果词典如下所示
print(result_dict)
{('Community A', 'Community A'): 1.0, ('Community A', 'Community B'): 0.6666666666666666, ('Community A', 'Community C'): 0.2, ('Community A', 'Community D'): 0.4, ('Community B', 'Community A'): 0.6666666666666666, ('Community B', 'Community B'): 1.0, ('Community B', 'Community C'): 0.0, ('Community B', 'Community D'): 0.2, ('Community C', 'Community A'): 0.2, ('Community C', 'Community B'): 0.0, ('Community C', 'Community C'): 1.0, ('Community C', 'Community D'): 0.75, ('Community D', 'Community A'): 0.4, ('Community D', 'Community B'): 0.2, ('Community D', 'Community C'): 0.75, ('Community D', 'Community D'): 1.0}
其中对角线元素显然是单位。
解释
get
函数检查是否已计算该对,否则它将执行计算
这篇关于在Python中计算多个词典上的Jaccard相似度?的文章就介绍到这了,希望我们推荐的答案对大家有所帮助,也希望大家多多支持编程学习网!
沃梦达教程
本文标题为:在Python中计算多个词典上的Jaccard相似度?
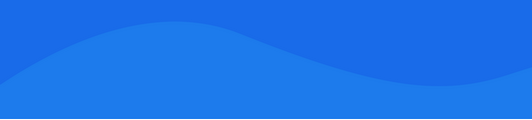

基础教程推荐
猜你喜欢
- 将 YAML 文件转换为 python dict 2022-01-01
- 症状类型错误:无法确定关系的真值 2022-01-01
- 哪些 Python 包提供独立的事件系统? 2022-01-01
- 合并具有多索引的两个数据帧 2022-01-01
- 使用Python匹配Stata加权xtil命令的确定方法? 2022-01-01
- 如何在Python中绘制多元函数? 2022-01-01
- Python 的 List 是如何实现的? 2022-01-01
- 使用 Google App Engine (Python) 将文件上传到 Google Cloud Storage 2022-01-01
- 如何在 Python 中检测文件是否为二进制(非文本)文 2022-01-01
- 使 Python 脚本在 Windows 上运行而不指定“.py";延期 2022-01-01