Fitting a sigmoid curve (Python)(拟合S型曲线(Python))
本文介绍了拟合S型曲线(Python)的处理方法,对大家解决问题具有一定的参考价值,需要的朋友们下面随着小编来一起学习吧!
问题描述
我正在尝试用S型曲线和三次多项式来拟合我的数据(成本与收入之比),然后找出拐点/收益递减的点。
这是我到目前为止拥有的代码,适应性不是很好。任何建议都将是非常有用的,谢谢!
import numpy as np
import matplotlib.pyplot as plt
from scipy.optimize import curve_fit
def sigmoid(x, a, b):
y = 1 / (1 + np.exp(-b*(x-a)))
return y
xdata = [ 404.91, 731.89, 804.23, 0. , 954.72, 954.72, 954.72, 744.54, 744.54, 498.5 , 355.03,
359.61, 0. , 0. , 0. , 753.77, 1116.02, 557.07, 589.06, 761.86, 722.97, 162.69,
354.47, 474. , 306.83, 538.57, 134.26, 134.26, 134.26, 134.26, 134.26, 652.29, 1296.26,
547.78, 845.22, 872.62, 881.59, 556.23, 500.2 , 569.97, 679.46, 679.46, 623.08, 628.33,
754.88, 2014.12, 1870.43, 1444.69, 826.05, 1071.03, 816.74]
ydata = [ 6986.97, 36591.27, 23702.95, 6380.01, 26873.68, 19398.27,24693.5 , 18435.52, 19066.1 , 8534.14, 8534.14, 8534.14,
2032.07, 567.26, 7544.64, 21051.07, 21051.07, 18592.84,18592.84, 18592.84, 19566.14, 4787.51, 7269.55,
11596.66, 9083.43, 13260.51, 6280.95, 4112.17, 6004.46, 7613.15, 6436.83, 10726.22, 20430.67, 8265.88,
15344.32, 30246.91,29928.96, 12215.02, 7776.27, 9714.94, 16642.3 , 29493.06,15496.04, 15496.04, 15496.04,
33397.61, 33397.61, 33397.61, 22525.93, 22525.93, 48941.98]
#fit 3rd order polynomial
p = np.poly1d(np.polyfit(x, y, 3))
second_deriv = p.deriv(2)
inflection = -second_deriv[0]/second_deriv[1]
print("polynomial inflection point:", inflection)
#fitting a sigmoid curve
popt, pcov = curve_fit(sigmoid, xdata, ydata, method='dogbox', p0=[1000, 0.6])
estimated_k, estimated_x0 = popt
print("sigmoid inflection point:", estimated_x0)
x = np.linspace(0, int(max(xdata)), len(ydata))
y = sigmoid(x, *popt)*max(ydata)
t = np.linspace(0, int(np.max(xdata)), int(np.max(xdata)))
plt.plot(xdata, ydata, 'o', label='data')
plt.plot(p(t), 'b-', label="polynomial")
plt.plot(x,y, label='sigmoid')
plt.legend(loc='best')
plt.show()
推荐答案
数据高度分散。根据所选的拟合标准(最小均方误差、最小均方相对误差或最小平均绝对误差等),可获得一致的结果。另外,对于常用的迭代方法软件,猜测方便的参数初值可能是不确定的。
所以,我站在这里不是为了回答你的问题,而是将你的结果与另一种非常不同和不同寻常的拟合方法的结果进行比较。该方法不需要迭代,也不需要参数的初始猜测。有关理论,请参阅https://fr.scribd.com/doc/14674814/Regressions-et-equations-integrales中的第37-38页这篇关于拟合S型曲线(Python)的文章就介绍到这了,希望我们推荐的答案对大家有所帮助,也希望大家多多支持编程学习网!
沃梦达教程
本文标题为:拟合S型曲线(Python)
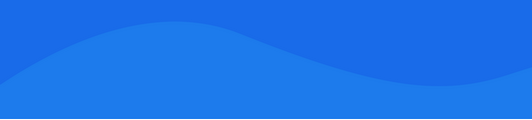

基础教程推荐
猜你喜欢
- 使 Python 脚本在 Windows 上运行而不指定“.py";延期 2022-01-01
- 哪些 Python 包提供独立的事件系统? 2022-01-01
- 如何在Python中绘制多元函数? 2022-01-01
- 使用 Google App Engine (Python) 将文件上传到 Google Cloud Storage 2022-01-01
- Python 的 List 是如何实现的? 2022-01-01
- 症状类型错误:无法确定关系的真值 2022-01-01
- 将 YAML 文件转换为 python dict 2022-01-01
- 合并具有多索引的两个数据帧 2022-01-01
- 使用Python匹配Stata加权xtil命令的确定方法? 2022-01-01
- 如何在 Python 中检测文件是否为二进制(非文本)文 2022-01-01